What Does The Function Mean In The Context Of Data Science?
Introduction
Here we explore the meaning of a function,
The Function
You recall that the output (also called dependent or response variables) is denoted using
You recall that the independent (or predictor, or feature, or input) variables, sometimes just called variables, are denoted by
One of the most important functions to remember in data science is
Here,
Refer to the graph below for a visual explanation.
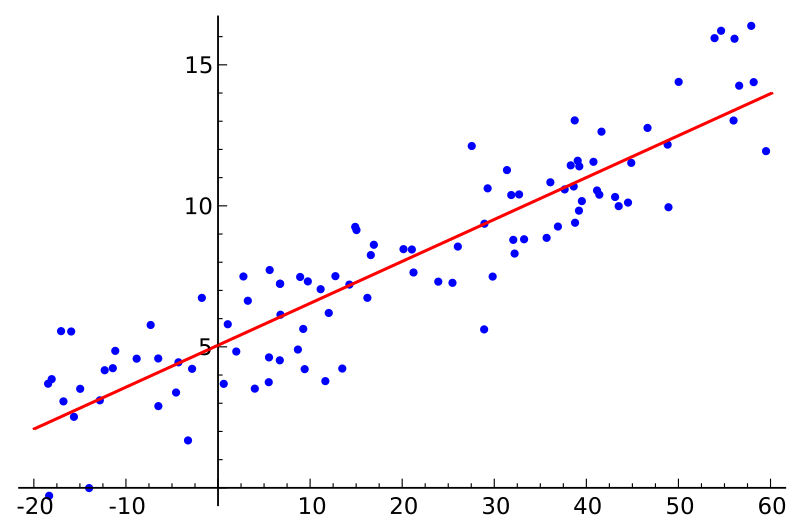
The blue dots are our
Motivation for Estimating
So far, we’ve declared that there is a fixed and unknown function
The trick is to create an estimation of what
In general, there are two things that we want from data: to predict and/or to infer. You can learn more about prediction and inference at the Prediction vs. Inference Starter Guide page. For our purposes, we are going to look at what estimating
Prediction
You will recall that a hat symbol (the ^ in
This says: "The estimation of
Reducibility of Error
But how accurate is
Reducible error is the error which we can improve by tweaking our analysis to better estimate
Mathematically, we can write this as
Where
is the average/expected value (
is the variance of the error term,
The goal of data science in general is to minimize the reducible error so that we can approximate f well enough.
How Do We Estimate ?
While there are many techniques to model data, they can be broadly summarized into two distinct approaches: parametric and non-parametric.
Recall that our goal is find a function
Let’s see how this is done using the parametric approach.
Estimating Using The Parametric Approach
We are going to present a 2-step approach in the form of OLS (Ordinary Least Squares). There are many parametric approaches, but OLS is one of the most common and you might already have seen it used (if you’ve done any kind of regression then you’ve used OLS).
1. We make some basic assumption about the shape of .
In OLS, we would start by modeling a linear equation that looks like
If you aren’t familiar with OLS, that’s ok; just know this is the standard form of an OLS equation.
You might recall that the
2. Now we fit, or train, the model.
Fitting entails using our training data to estimate the parameters.
In the case of OLS, finding the
Estimating Using The Non-Parametric Approach
In our previous example of OLS using the parametric approach, we had to assume that
The non-parametric approach avoids the question of assuming what kind of function
Refer to the image below for an example of a non-parametric technique, called splines.
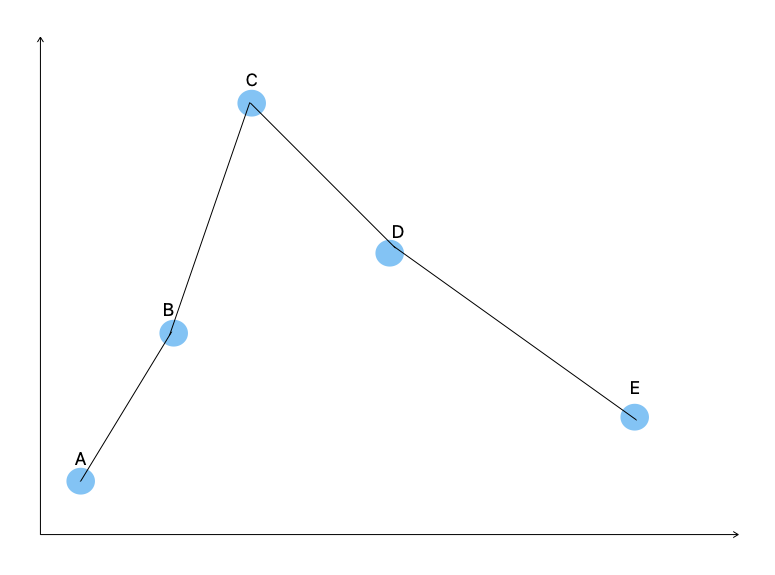
The above image is a spline fitted to 5 data points. You don’t need to know what a spline is, other than that it creates a line from point A to point B, point B to point C, etc. This makes it non-parametric; it merely creates a (typically) polynomial equation in a piecewise fashion (in this case, the black line which is our
To see the downsides of non-parametric approaches, imagine that we got one more random data point, marked by the green dot.
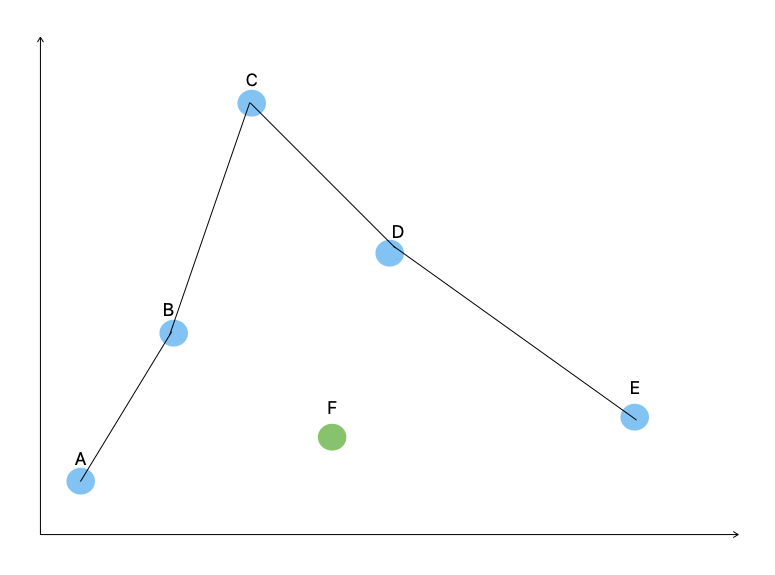
Our
This is an example of one of the disadvantages of non-parametric approaches: although they fit the data with a reducible error term of 0, when encountering new data they can be fairly off when trained on small amounts of data. In our example of 5+1 data points, we have very little data and a relatively simple polynomial equation to represent it. But what if we had millions of data points in
Our Sources
-
www.statlearning.com, chapter 2.1.1